This community is in archive. Visit community.xprize.org for the current XPRIZE Community.
Major Constraints in regard to Frontline Health Systems in LMICs
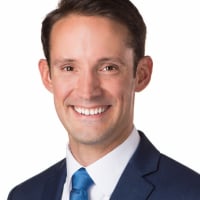
Over the past decade, discussion of integrated care has become more widespread and prominent in both high- and low-income health care systems (LMICs). The trend reflects the mismatch between an increasing burden of chronic disease and local health care systems.
Healthcare challenges in LMICs have been the focus of many digital initiatives that have aimed to improve both access to healthcare and the quality of healthcare delivery.
Healthcare challenges in LMICs have been the focus of many digital initiatives that have aimed to improve both access to healthcare and the quality of healthcare delivery.
- What are the biggest constraints when considering frontline health systems in low- and middle-income countries?
- Which aspects of these systems require the most work?
- How can they benefit from advances in A.I. technology?
2
Comments
Advances in clinical practice that gain efficiencies are among the few ways to simultaneously improve all ends in the “iron triangle” of healthcare quality, cost, and access. Most advances will otherwise cause trade offs or increase disparities that impact underserved populations, for there is no quality without access.
Electronic consultation systems allow clinicians to request specialty advice through messages, teleconferencing, or online crowdsourcing. These illustrate the potential to treat patients with up to 71% fewer in-person specialist visits. This eliminates patient time and travel to initiate a treatment plan (days vs. months), which could be lost completely when up to 40% of specialty referral requests are not completed. A recent literature review of such systems found improvements in process measures such as timely access to care across a range of studies, but also noted the need for further research on how best to design such systems to ensure improvements in clinical outcomes. Despite benefiting patients and providers, existing (electronic) consult systems remain fundamentally constrained by the availability of human experts.
In the face of ever escalating complexity in medicine, integrating informatics solutions is the only credible approach to systematically address challenges in healthcare. Tapping into real-world clinical data streams like electronic medical records with machine learning and data analytics will reveal the community's latent knowledge in a reproducible form. Delivering this back to clinicians, patients, and healthcare systems as clinical decision support will uniquely close the loop on a continuously learning health system. Our research group seeks to empower individuals with the collective experience of the many, combining human and artificial intelligence approaches to medicine that will deliver better care than what either can do alone.
Therefore, I believe that the biggest constraints are dependence on medical providers in LMIC and lack of task-shifting. Several models of task-shifting have been developed and need further validation. One example is the SEVAK model in Gujarat, India (www.sevakproject.org) which utilizes village level high school students to train them in measuring and following hypertension and diabetes, by making life-style changes rather than reliance on medications. This model was also tested in Guyana, South America, with initial success (https://academic.oup.com/milmed/article/180/12/1205/4160666).
Hi @Lizzy_2020, @PHall, @acavaco and @marschenrj - You may have thoughts on the major constraints to Frontline Health Systems. Join the discussion to share your thoughts. Thanks.
Hello @gajewski, @angelfoster, @Budoff, @timothymusila and @andwhite - It would be great to hear your views on the major constraints to FLH systems. Please join the discussion.
Hi @Shabbir - We would love to hear your thoughts on how exponential technology can help resolve all/few of the constraints listed above.
@RahulJindal thank you for your thoughts about Project ECHO. Task-shifting is core to the ECHO Model, and exactly what we do to build capacity amongst frontline health workers in low-and-middle-income countries. By democratizing knowledge - bringing the right knowledge to the right place, at the right time, we build the capacity amongst healthcare workers, shifting what has traditionally been done by doctors to nurses, nurse practitioners, Community Health Workers, etc. Learning effective triaging, deciding who needs to see a physician and who could be well-served by a Community Health Worker, is part of this knowledge. By partnering with The WHO, the CDC, Ministries of Health, and other on-the-ground experts, we are able to build local capacity.
Unlike other healthcare workforce “pipeline” training models where knowledge only flows one way, the ECHO model is a platform that allows all user-participants to co-create knowledge through group discussion, peer interaction, and engagement of local expertise so that all teach and all learn, and our collective understanding of how to disseminate and implement best practices across diverse economic and cultural contexts grows.
I also wonder who decides "bringing the right knowledge to the right place, at the right time" for a community worker in a remote village in India, Africa or South America? Who makes a decision on what is correct methodology or treatment pathway? Should we in the USA make such decisions?
Artificial intelligence could potentially factor in by allowing the community worker ask questions and interact with ie. Amazon's Alexa or google. I envisage an 'open source' fund of knowledge which is not under the domain of a university, Federal agency or a government.
So, two problems we can look into:
1. Rapid dissemination of latest guideline and best practices does not happen normally. How ca AI help remove this bottleneck?
2. Real-time clinical collaboration across hospitals and countries does not happen normally because too much resources require and international interoperability of clinical data is difficult. Is there new AI solution for this?
XPrize has formed a covid pandemic alliance. The alliance is probably also looking at the major constraints on covid patient care in LMICs, such as the lack of rapid dissemination and real-time clinical collaborations in current global clinical infrastructure. If we can have some cross-talk, that may help identify the constraints amplified in covid pandemic.
@ajchenx - We would like to read more about the AI-powered Internet-based primary care team model you'll have designed. Is it possible for you to share link to this resource.
If we can reason from there, we can realise there are hundreds if not thousands of constraints with both the current sickcare and the prospective healthcare. Now the right question I think we need to ask is, what would we like to solve with the current sickcare regime and what would we like to solve with the healthcare system.
We might want to categories the process as follows, with obvious overlaps:
Healthcare
1. Health Insurance.
2. Diet
3. Exercise.
4. Screening.
5. Vaccination.
6. Mental Health.
Sickcare
1. Administration (personal health records).
2. Diagnostics.
3. Treatment.
4. Medication.
5. Surgical.
6. Rehabilitation.
And so on and so forth!
And lastly, across-board "data" format, accessibility and interoperability is a huge problem.
Which aspects of these systems require the most work?
Now that I am beginning to think about this, an aspect that would require the most work is creating a healthcare system that is aligned to local needs, because the population is so diverse, even if we tend to think of, for example, Mexico, as a unique, homogenous mass of people, diseases are diverse and dependent on regional, cultural, and social environments.
How can they benefit from advances in A.I. technology?
AI technology can be used to include the social factor in healthcare system designs, especially with respect to data collection and analysis.