This community is in archive. Visit community.xprize.org for the current XPRIZE Community.
Options
Developing the Foundation for Healthcare Data Integration
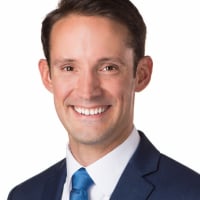
in Prize Design
In many countries, patient data resides across multiple point-of-care systems, and in a variety of structured and unstructured formats. Often, various point-of-care systems (clinics, primary physicians, hospitals, pharmacies, etc.) within a health system collect the same information and can’t share that data with other point-of-care systems, leading to an inefficient system and a fragmented picture of a patient’s health.
Breaking down the barriers to data access while adhering to privacy and security principles is an immense challenge and tremendous opportunity. Standardized and granular patient data that can be shared across systems provides the foundation for a low-cost and high-quality healthcare, including through enabling frontline health systems to function optimally and effectively. Additionally, such data is essential for AI algorithms to deliver insights.
In such a scenario, data standardization and aggregation is top priority. We want to know:
Breaking down the barriers to data access while adhering to privacy and security principles is an immense challenge and tremendous opportunity. Standardized and granular patient data that can be shared across systems provides the foundation for a low-cost and high-quality healthcare, including through enabling frontline health systems to function optimally and effectively. Additionally, such data is essential for AI algorithms to deliver insights.
In such a scenario, data standardization and aggregation is top priority. We want to know:
- What are the measurements of successful data standardization and aggregation, or systems interoperability? How has it been assessed in other sectors or industries, such as finance?
- What is the best analog for this type of data standardization and aggregation from another sector or industry?
- What technologies can help with data standardization and aggregation? How can AI and ML help?
- What considerations need to be made with respect to an infrastructure that still protects privacy and security?
1
Comments
IMHO, ML/AI role is in extracting knowledge from data lakes/ocean. Humans need to set the standards for content structure and interfaces (like was done with many "Omics").
Hello @aylin, @sarahb, @sarahkhenry, @ukarvind, @ingmarweber, @KarenBett, @Pavel - This discussion is related to one of the other prizes we're designing: Frontline Health. I know you have expertise in technology specifically AI. I wonder if you have any suggestion on data standardization and aggregation across various platforms? Any analogs from a different sector / industry on data standardization and aggregation would also be helpful in understanding this topic.
1. https://port.oceanprotocol.com/t/dataset-structure-formatting-standards/50?u=bngejane.
2. https://oceanprotocol.com/technology/compute-to-data
I think the approach that Ocean has embarked on can address some of the issues raised here.
Hi @Shabbir, @jda @fbaothman @acowlagi @elekaja @MachineGenes @Nitesh @yuanluo @dykki @kenjisuzuki @SArora - As a tech expert in digital health space, you might have inputs to share on data standardization and aggregation. Please join the discussion.
This system has worked only in the single caregiver system like public health or hospital chain. To enable a cross caregiver data transfer there has be a partnership like partners in health in Boston.
For the first we can start work in a public Heathcare system of a state/county/district to prove a model and showcase it's benefit to local administrators for a fulltime integration in to a larger system.
For the data security and privacy, there are already many standards available like HIPPA compliance which can be but into the data collection system.
Thank you for the questions and reactions. I have the impression that a question like "Which kind of Data is the most important to aggregate to make better medical research?" could be added, but maybe it is another topic.
Cheers. Didier
Hi @cimdal2, @preciouslunga, @owen, @Debbie_Rogers, @biki, @sakeuriticus, @lswright, @CHardaker, @Hongsoo, @care2communities, @kkatara, @alabriqu, @abejanis, @ShmuleyG and @rguimara - As you are tech expert and entrepreneur, we feel you might have inputs to share with us on the technologies which can help us with data standardization and aggregation. Join the discussion.
Since the question is so important (and also so big), how does a country come to answer that question? What key perspectives are needed?
The Community Data Working Group developed a toolkit which integrates and builds upon various tools and methods (historical and current) designed to assess data quality at facility level, taking into account good practices and lessons learned from many country contexts.Module 1 looks at Framework and Metrics, Module 2 looks at a Desk Review of Data Quality and Module 3 looks at Data Verification and System Assessment.
The World Bank ID4D group developed A Catalog of Technical Standards for Digital Identification Systems, which includes Technical Standards and an overview of standards setting bodies, Frameworks for Interoperability which is interesting to review. The application to health is captured in The Role of Digital Identification for Healthcare : The Emerging Use Cases document.
Considerations with respect to infrastructure which protects privacy and security:
1. Legal and policy frameworks must be in place first and foremost. secondly, the technology must be appropriate for the entire country context, not just the city center, but can work on and offline in places with limited or no connectivity and unreliable energy sources.
We have started exploring future use cases for AI and ML in healthcare. If you have inputs / experiences on it, please share it here.