This community is in archive. Visit community.xprize.org for the current XPRIZE Community.
Use cases for AI and ML in healthcare
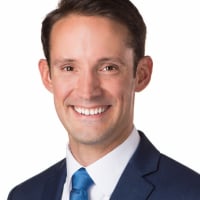
in Prize Design
We are exploring future use cases for AI and ML in healthcare. Imagine all things are possible.
- What are some of the future use cases for AI and ML in healthcare?
- How can AI/ML be leveraged for population health management?
- How can Al/ML be leveraged for personal health?
- How can AI/ML be used to evaluate health programs?
0
Comments
1. Use AI/ML to study patient journey from EHR data, build doctor referral and symptom checking AI model, test automatic doctor referral in big hospital workflow. Goal: improve hospital patient navigation efficiency and accuracy.
2. Use the doctor referral AI tool in rural clinical to enable rural doctors to refer patient to the right specialties. Goal: reduce health care disparities in rural areas.
3. Use AI/ML to automate guideline recommendation, patient matching and patient education for enabling real-time clinical collaboration cross hospitals. Goal: reduce health care disparities in developing countries.
4. Use AI/ML to discover digital biomarkers for preventive screening for cancers, neurological diseases to improve population health.
Hi @jda, @aassif_lg, @meallen3, @fbaothman, @scveena, @synhodo, @mashizaq, @Sujana, @dzera and @skornik - We look forward to your thoughts on future use cases for AI and ML in healthcare.
Another application of AI that is likely to transform the health sector is the Dictation Assistance using (Natural language Processing) NLP and Translation. Natural language processing can help medical experts deployed in remote areas to capture data from dictated notes, translate and store in the Electronic Health Records (EHR) despite language barriers. NLP can also help in sorting unstructured patient data including images and text to extract vital information for treatment.
AI will also help bridge the existing gap between the number of surgeons and patients. AI will assist experts in diagnostic processes by analyzing medical images like CT scans, X-rays, and MRIs. Through imaging analysis, medical experts will extract important information that may not be possible through human eyes. AI has also been applied in the field of oncology to help in cancer treatment. For instance, IBM Watson is used in processing unstructured and structured patient data to provide cancer treatment recommendations.
Hi @SArora and @RahulJindal - What are your thoughts on the future use cases of AI and ML in healthcare?
Hey @mhackett, @stephaniel, @dollendorf, @JoanneP, @siimsaare, @abejanis, @Lizzy_2020, @Ewunate, @ArdenVent, @kevinnicholasbaker, @berniceredley - Curious to know if you have any inputs on the future use cases of AI and ML in healthcare.
Accessing available data on cost-effectiveness of health interventions to create a locally-relevant profile and developing predictive models
Hi @DidierC, @janansmith, @ClaireM, @mendezra2, @marschenrj, @RickyM, @clairecravero, @acavaco, @care2communities and @C_Castellaz - What do you think on the way AI and ML will be used in the future for population health management and evaluating health programs.
@Shashi AI and ML, as @synhodo shared, have the potential for huge impact in LMIC’s for disease screening and diagnosis, that leads to early detection and provides access to quality healthcare for rural and underserved communities. One of the biggest challenges to clinician training is the exponential growth of medical knowledge, which currently doubles every few months. AI can help aggregate this knowledge and get it to where it’s needed most. We at Project ECHO see AI playing an increasingly important role in this respect, and in combination with the ECHO Model, helping to democratize knowledge, get it to where it’s needed most, and save lives.
https://www.businessinsider.com/amwell-bests-telehealth-competitors-on-user-satisfaction-2020-10?IR=T
https://africanews.space/africa-needs-telemedicine-to-overcome-its-medical-challenges/?fbclid=IwAR39VRIFmDmrY2We2Dk4wlJW3Q2pvBu1C0RhtjzjcMpk6yU3Kwf5eGudpxI
Hey @Shashi, we're using new forms of evolutionary ML and adversarial AI-- not Generative Adversarial Networks (GANs), but a completely different form of adversarial AI we developed a couple of years before GANs, which has significant operational advantages over the status quo.
Conventional ML is based on artificial neural networks (ANN), which suffer from inherent limitations (the main one being that they never really explicitly 'model' the dynamics of a system, but merely come to 'embody' those dynamics of the system). In contrast, our form of evolutionary ML takes the best abstract mathematical models of a disease from the medical literature, including its dynamics-- the way it alters the function of the body, and interacts with drugs--and embodies this structure as simulated "chromosomes". Every "gene" on the chromosome corresponds with some parameter associated with the disease or drug-disease interaction. We then take individual medical histories and use evolutionary pressures to force the chromosomes to evolve-- to select and breed and mutate, across generations-- until organ-scale personalized models have been produced that embody the disease dynamics in the individual subject. (This is an idea that has been around for decades in a form originally known as "genetic algorithms", GAs, but GAs had fundamental limitations that prevented their real-world use for complex diseases and partial information. By re-thinking models of evolution and adding additional features we've solved those limitations, and so now can apply our modified evolutionary process to model complex real-world diseases.)
One of the problems that remains is ambiguity in the evolved computational models. We use a novel adversarial process, whereby the main AI has two subordinate AIs take those models and play a game with them. One subsidiary AI, the "Prime", takes the models and uses them to try to design an appropriate drug therapy. The other AI, the "Adversary", uses known ambiguities within the models to cause adverse outcomes, which the Prime then has to counteract by modifying its strategy. (Unlike ANN, our AI "knows what it knows", including ambiguities among the chromosomes.) The result is ultimately generation of a drug dosage strategy that is personalized, and has been tested for safety and efficacy. This adversarial process was first demonstrated in 2012 and patented in mid-2014, six months prior to GANs. It has major advantages over existing AI-based techniques, in that it is completely explainable, interactive with the users (patient and clinician), able to generate good strategies despite a training history of therapeutic failures, and is able to operate the AI component on an isolated Edge device.
For the IBM Watson AI XPRIZE we demonstrated this technology to generate insulin strategies for highly-unstable forms of type-1 diabetes by data-mining actual medical histories, despite those histories being comprised solely of therapeutic failures to achieve the desired blood glucose outcomes. Last year, as part of AI XPRIZE, we demonstrated our adversarial AI running on an isolated laptop computer, generating 30 hours of insulin strategy over approximately 20 minutes of computing time, that generated far better blood glucose outcomes than the original histories. The generated insulin strategies look nothing like conventional insulin dosing.
This suggests that the medical use of AI is about to look very different from previous use cases.