This community is in archive. Visit community.xprize.org for the current XPRIZE Community.
Which gender data gaps are the most important to address first?
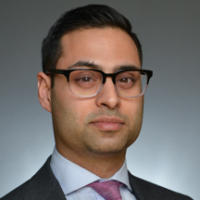
In Invisible Women, Caroline Criado illustrates that in multiple industries, vast amounts of data on women is incomplete or missing — despite a global population that is half female — resulting in a massive gender data gap. The implications of this assumption range from small inconveniences to matters of life and death.
Whether it be missing gender data in the areas of health, education, economic opportunities, politics, or security — we want to know: which gender data gaps are the most pressing, and why?
Whether it be missing gender data in the areas of health, education, economic opportunities, politics, or security — we want to know: which gender data gaps are the most pressing, and why?
2
Comments
Data2x is an organization working to improve the quality, availability, and use of gender data in order to make a practical difference in the lives of women and girls worldwide.
In a recent study, they identified need, population, and policy relevance as three criteria when prioritizing data gaps to map.
Within each of the domains of health, education, economic opportunities, politics, and security, I'm curious to know what our online community of experts feels are the most critical gaps to close first, and why?
If we look within the domain of health, for example, is it more important to focus on closing data gaps in mental health, adolescent health, disease burden, or another area? Why?
The FDA acknowledges the efficacy and safety of drugs can vary between men and women (https://www.fda.gov/science-research/womens-health-research/understanding-sex-differences-fda). I'm curious to know more about the gender data gap issues in pharmaceuticals. Thoughts?
Register here: https://lnkd.in/e-iXRkk
Looking forward to extract important insights from this webinar.
I think so, too. Maybe this young EU project is relevant in this regard: https://www.granted-project.eu/home/
While it is hard to prioritize what gaps to fill first, some broad principles that we believe should are important to consider when filling a gap are:
1. Inclusiveness. We need to count people who are currently marginalized in order to understand their needs and develop and implement adequate programs and policies.
2. Intersectionality. If it isn't being adequately captured with pure data, shifting the conversation towards what we like to call “knowledge gaps” rather than “data gaps”. How does x affect y? (to capture the impact of various factors on women’s lives). Qualitative data is so important but is based on small samples (not generalization nor representative). How can we scale up collection of qual data that really gets to the lived realities of girls and women?
3. Impact. In particular, gaps related to long-term impacts and cost-benefit analysis. So much of current data collected is cross-sectional. Evidence that builds the case for gender equality and shows how women’s full participation in socio-economic and political spheres reaps benefits not just for themselves, but their families and societies as a whole is really helpful.
4. Scalability. What works to advance gender equality? There are so many smaller-scale studies of great practices or programs that have worked here and there, how can we move some of these to be acknowledged as high-impact practices worldwide?
5. If we want to focus on gender in the SDGs, the lack of data on gender-specific indicators is alarming: "only 12 of the 54 gender-specific indicators on the SDGs, or 22%, have enough data that is regularly produced and available that can be used to monitor progress across all regions" according to UN Women. We need this data to keep governments accountable.
When women are not accurately represented in datasets, for example the health domain, the analysis about them, especially if it is an algorithmic process usually ends up being inaccurate and in some cases completely wrong since different social groups have different patterns. AI algorithms are optimized to learn the patterns of the majority group unless they are explicitly presented data from different social groups. Consequently, for example an algorithm on cardiac disease would learn the symptoms and patterns associated with (white) men and potentially lead to catastrophic results for women as well as other non-majority groups.
@Andrea I agree that the most disruptive, innovative and powerful thing we could do would be to develop something that would help data custodians understand, collect and analyse data with an intersectional lens.
A couple of other - off the top of head thoughts on gender data gaps and areas of need - although I will go and do some further thinking:
- mobile technology - I understand that the impact of 5G has only been tested on men and not of its impact on women or children
- violence against women - we have some public data on prevalence, incidence and response but very little on early intervention and recovery. It would be great to have this on the radar. Also it would be revolutionary to encourage or incentive private companies to share their sexual harassment and sexual assault data (de-identified of course).
- we must also include in our thinking gender non-binary as well as male and female identified genders. There is virtually no data on gender-non binary
- mental health - this is front of mind for me as we go through a global pandemic and there is a Royal Commission into Mental Health in Australia. The interim report on mental health had very little on gender and mental health even though it presents differently and at different rates depending on gender
- AI - my understanding that biases have been built into algorithms that underpin AI (see the google translate example that I think is in Caroline's book) as women are not part of the teams in the development and testing of AI
Thanks
@aylin Are you working within social media platforms? There is a TON of discussion about chronic pain management and many women who are feeling abandoned by traditional medicine.