This community is in archive. Visit community.xprize.org for the current XPRIZE Community.
Core Problems of Gender Data Gap Challenge
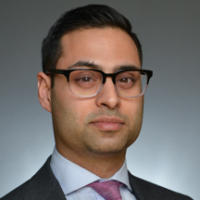
We organized gender data gap challenges in health into a set of core problems. Each core problem reflects a subset of related issues central to the gender data gap challenge. From unconscious bias to data accessibility, these problems are barriers that prevent gendered data from being produced, used, and applied. Each of these core problems is an umbrella for several sub-issues, and many of these core problems intersect with each other. One core problem can also lead to the other problems. Below, we have provided the six core problems:
Have we missed any core problems? Are there any other significant problems which hinder gendered data collection? What innovations are addressing these core problems?
- Social norms, patriarchal structures, and bias render gendered data invisible or ignored
- Gender data is not collected consistently across domains
- Lack of granularity of data across domains / data is not disaggregated
- Accessibility of data is restricted due to privacy and sensitivity concerns
- Data are under or mis-analyzed
- Data is not applied to policy and practice, and accountability systems are not in place to ensure that data is appropriately used
Have we missed any core problems? Are there any other significant problems which hinder gendered data collection? What innovations are addressing these core problems?
4
Comments
Do you'll have any insight on Karan's questions? You can share with the community. Thanks.
No consent means that now, when we need to mobilize the population for cures and stratify the population for risk, we have worthless de-identified genomes to work with. To deploy consented genomes at scale is super easy, dirt cheap, and can be done by reusing mapping software hospitals, community clinics and governments have already have paid for.
Short answer. VC's and NIH keep funding to men from MIT and the Broad who don't want to be bothered with consent.
If you want a foundation for AI and ML to work with health data, try funding women that build solutions for consenting genomes at a global scale
Women are not believed when it comes to degree of pain or symptoms, with these being attributed to "hysteria" or feminine weakness, when the opposite is more often true.
Test results for women are often not believed and this results in re-testing for the same attributes. I have seen multiple x-ray, CT Scans and ultrasound investigations for an ultimate diagnosis of Endometriosis, which can only be diagnosed via laparoscopy.
Diagnosis is taught in a male oriented frame where anatomical differences are glossed over.
Most of this is medical professional attitude, being that in the teacher or the student.
This generates a lack of trust and therefore data can be withheld due to that lack of trust, resulting in data pools that are gender biased, reinforcing the sterotypes.
7. The behavioral economics of the exchange (information for services) are not often in the women's self-interest.
Many data collection initiatives never stop to consider why a woman would *want* to share her data? (And not some feel good social justice reason, but true self interested reason)
For men, when there are so many drugs and solutions under research and brought to market, the answer is pretty easy: sharing information = solving their problem. The trust issue also plays a role here.
Also on issues of violence against women, reported crime data is very inadequate in many countries as we know there is tremendous under reporting. The DHS kind of survey in the UK is a model that provides victimisation data. In India we have a similar one called the National Family Health Survey which focuses on health indicators but also domestic and intimate partner violence. We need to look at other forms as well.
It is important to directly measure those characteristics. As an example, think of exoskeletons. The application of exoskeletons depends on strengths and body measurements of its users but not on their sex. In practice, due to bias in design processes, the exoskeletons are regularly optimized for the average male user. So: sex and also other diversity characteristics should be treated as proxies and it should be tried to measure their effects more directly. However, sex data needs to be collected to learn more about its correlations with the more direct variables.
Thanks.