This community is in archive. Visit community.xprize.org for the current XPRIZE Community.
Evaluating Quality of Data Collected by Teams
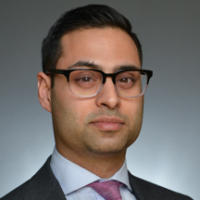
Four core problems identified by the design team are related to data integrity and usefulness as follows:
We want to learn from you:
- Data is not disaggregated and lacks granularity for analysis
- When data is collected, it may be under or mis-analyzed
- Data accessibility may be restricted due to privacy and sensitivity concerns
- Once collected, data is not always applied to policy and practice.
We want to learn from you:
- Data2X identified how the desirable features of gender data include quality (valid and reliable sources), coverage, and comparability (of concepts and measures).
What other desirable data features would you prioritize? - New data collection methods, such as using apps or social media, often attempt to engage users and capture their attention in unique ways. Validating these forms of data and the insights they provide can be a challenge.
As researchers increasingly use big data from social media and apps to detect mental health problems, how have they assessed the validity of these data points and measurements?
1
Comments
Let us look at a concrete situation. "Women in nonwestern societies are often the victims of violence. It may be in the form of wife beating or assault. It may be in the form of genital mutilation. It may be in the form of bound feet or other painful actions that are related to changes in status as for example when a female is ready for marriage, or can resume sex after her menses, etc. We can of course collect data on this violence. We can get reliable data on the physical damage caused by the violence. But unless we can substitute less violent behaviors we cannot end the violence and the data collection is of no value.
Let us illustrate the point in the case of bound feet. Bound feet of course is associated with ancient China. Young females had their feet bound to make them more desirable as mates. Who supported this painful and perhaps humiliating custom? The matriarchs were big supporters. The family matriarchs wanted grandchildren or great grand children. They wanted their daughters to find desirable husbands. To western minds, bound feet were brutal and did nothing to make the women of China more attractive. But bound feet probably disappeared in China as much because of the fact that a one child policy made females more desirable simply because they were rarer in a society that still practiced female infanticide into the present time(and it may persist in some isolated rural communities). In short, we substituted other qualities of a woman,(and that made her attractive (including the simple mechanism of having fewer of them for men to select) for the violence of binding feet. We must take the same approach of finding less violent behaviors that fulfill the valid purpose of the violence with other forms of violence that undoubtedly have mental health consequences such as depression on women.
@karenbett, @qlong, @DrLiliaGiugni, @EVSwanson, @NielsRU - As a researcher, we feel you all may have knowledge on assessing the validity of data points and measurements for data collected from social media and apps. It would be great hear your experience. Join the discussion. Thanks.
Note that in our work noise, such as users lying about their characteristics or fake accounts are, per se, not an issue as long as their percentage is somehow consistent. What is more of a challenge are patterns that cannot be modeled, such as lots of fake accounts in one country, and almost none in another. Those would then create challenges to correct for.
Other researchers in other domains validate data at the individual level but, typically, we don't have individual level ground truth and can't apply that methodology.
What are the challenges that you see emerge most often when validating data at the individual level? What emerging tech do you see as having the potential to mitigate those challenges?
Hi @MarioCardanoItaly and @mab - Please share your thoughts on assessing validity and measurement of social media data points. Thanks.
Complexity here means the ability of datasets to deliver insight across a variety of domains. Since this often not the case, we need the data we do have to be as interoperable as possible in order to combine and link different datasets to get the levels of analysis right and deliver insight. For this to work, datasets must be amenable to being joined, aggregated, split, and cross-tabulated, in short, fit-for-purpose. This will both be a function of dataset construction (unit of analysis, available group variables, etc.) as well as dataset dissemination (formats such as online tables, downloadable pdfs, csv, APIs, etc.)
Also if you have any experience in assessing the validity of data points and measurements of data collected from social media and apps, please share it with us.
A data quality checklist might also evaluate usability (is the data understandable, accessible, etc.), flexibility (how can it be manipulated and compared across datasets), privacy (who can access it, when and how), and value (cost/benefit analysis, relevance to mission).
- We conducted onsite visits to observe client sessions and how case workers entered data into the system.
- We spoke to case workers to see if they had a clear and consistent understanding of how data should be captured and how would be used.
- While onsite, we also spoke to clients in care.
- We conducted manual reviews of the data being collected and sense checked linkages between records, looked for anomalies, etc.
- We noted any discoveries and discussed with subject matter experts to see if the units we visited and what we observed were representative.
This approach was far from comprehensive but did reveal some useful insights into the validity and accuracy of the data.